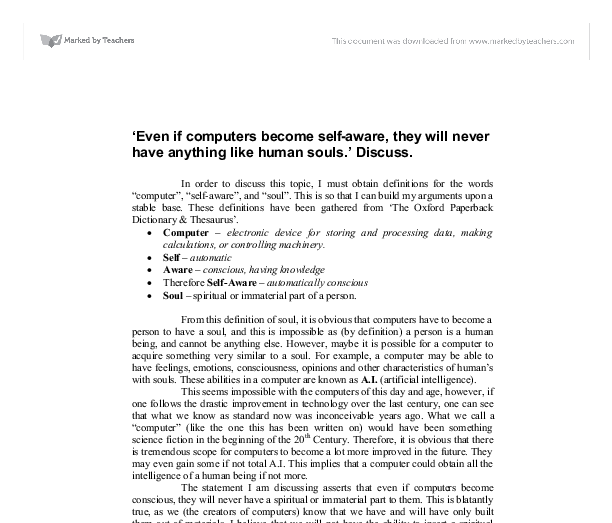
This article will examine Deep Learning's limitations, and the opinions expressed by Experts. It also explores possible solutions. These limitations include the cost and time required for labeling and collecting data. Deep Learning is not to criticized. It should be viewed instead as a discussion on the limitations and potential of this emerging technology.
Experts' views on deep learning limitations
Deep learning requires large amounts of data to be able to learn. Deep learning algorithms can perform poorly when the data volumes are small. Standard machine-learning techniques are capable of improving performance without massive data volumes. To overcome these limitations, deep learning techniques need to be coupled with unsupervised learning methods, which do not rely heavily on labeled training data.
Deep learning algorithms make use of multiple layers for training computer programs. Each layer uses learned information to create statistical models. This process continues until the output is acceptable in accuracy. The number processing layers in the algorithm is the basis of the term "deep".
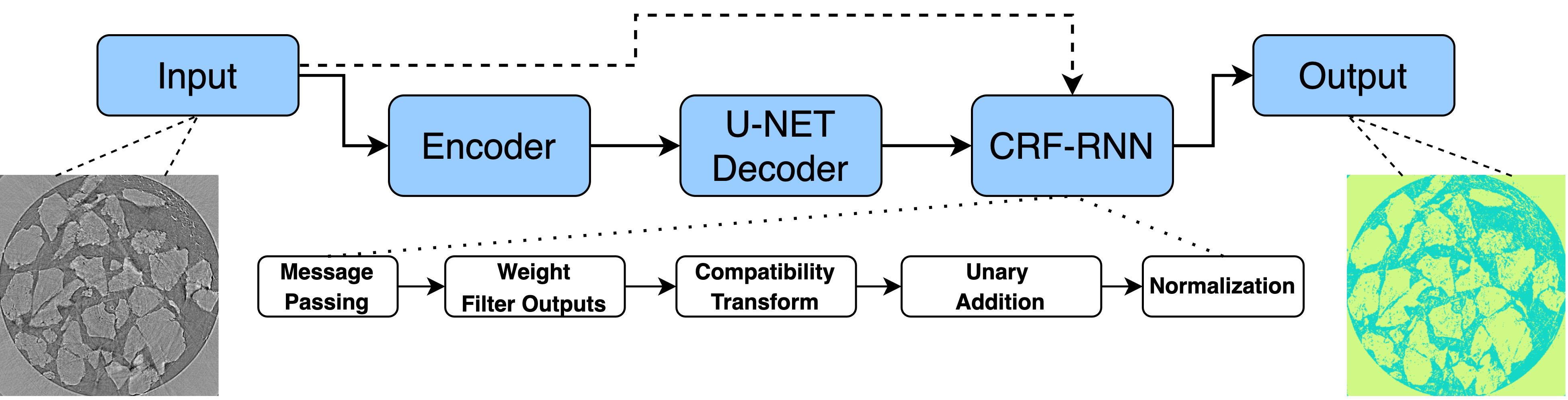
Deep learning requires a lot of processing power, in addition to the data needed for programs. However, if you have a large number of unlabeled data, deep learning programming can create complex statistical models directly from the iterative output. These devices produce huge amounts of data that are not labeled, and the internet of things is making it more popular.
There are solutions
Deep learning offers many potential benefits but it also has its limitations. It lacks the necessary training data to be able perform classification tasks. Furthermore, it cannot solve problems requiring reinforcement learning or rule-based programming. These limitations are being overcome by some AI researchers who focus on neuroscience.
Deep learning is a very powerful method of learning. It relies on very few human inputs. This means that it needs a lot more data and computing power. But with the right infrastructure, high-performance GPUs, and the right training tools, training time can often be drastically reduced. Deep learning models are much faster than human operators. Furthermore, their quality never decreases as the training dataset grows.
While deep learning may still be in its infancy stages, it has been a huge success in many areas. One of its most promising applications is gene expression prediction. A deep neural net with three hidden layers has proven to be more effective than other methods such as linear regression. These methods can also be clinically useful, since they can use fluorescence microscopy to identify cellular states.
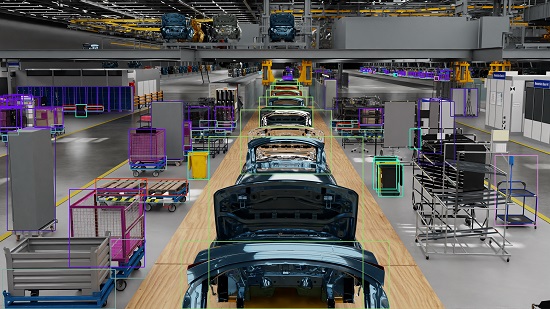
Time and cost requirements for collecting and labeling data
Collecting and labeling data for deep learning models can be costly and time-consuming. Open-source datasets can be difficult to label. Experts are a good option. Experts such as these are highly paid, and they dedicate a lot time to the task. They can also extend the deadline due to their high costs. Moreover, it is costly to hire new labelers to scale the workforce.
Crowdsourcing is another option to label data. It's also cost-effective. You can set up a reward for each assignment. For example, a $100 reward could be set for labeling 2000 images. For that price, you can complete up to nine assignments. Crowdsourcing, however, is not ideal since the datasets may not be high quality.
Not only is it important to label data, but also the preparation and storage of them are major costs. Annotating videos is an extremely labor-intensive process. A 10-minute video containing 18,000-36,000 frames, with a frame rate of 30-60 frames per second, requires more than 800 hours of human labor.
FAQ
What can you do with AI?
AI can be used for two main purposes:
* Prediction - AI systems can predict future events. A self-driving vehicle can, for example, use AI to spot traffic lights and then stop at them.
* Decision making - Artificial intelligence systems can take decisions for us. For example, your phone can recognize faces and suggest friends call.
What does the future hold for AI?
The future of artificial intelligence (AI) lies not in building machines that are smarter than us but rather in creating systems that learn from experience and improve themselves over time.
In other words, we need to build machines that learn how to learn.
This would enable us to create algorithms that teach each other through example.
Also, we should consider designing our own learning algorithms.
Most importantly, they must be able to adapt to any situation.
How will AI affect your job?
AI will eliminate certain jobs. This includes truck drivers, taxi drivers and cashiers.
AI will create new jobs. This includes data scientists, project managers, data analysts, product designers, marketing specialists, and business analysts.
AI will make existing jobs much easier. This includes accountants, lawyers as well doctors, nurses, teachers, and engineers.
AI will make jobs easier. This applies to salespeople, customer service representatives, call center agents, and other jobs.
Why is AI so important?
It is estimated that within 30 years, we will have trillions of devices connected to the internet. These devices will include everything, from fridges to cars. The Internet of Things is made up of billions of connected devices and the internet. IoT devices will be able to communicate and share information with each other. They will also be capable of making their own decisions. For example, a fridge might decide whether to order more milk based on past consumption patterns.
It is anticipated that by 2025, there will have been 50 billion IoT device. This is a huge opportunity to businesses. It also raises concerns about privacy and security.
Statistics
- In 2019, AI adoption among large companies increased by 47% compared to 2018, according to the latest Artificial IntelligenceIndex report. (marsner.com)
- The company's AI team trained an image recognition model to 85 percent accuracy using billions of public Instagram photos tagged with hashtags. (builtin.com)
- In the first half of 2017, the company discovered and banned 300,000 terrorist-linked accounts, 95 percent of which were found by non-human, artificially intelligent machines. (builtin.com)
- A 2021 Pew Research survey revealed that 37 percent of respondents who are more concerned than excited about AI had concerns including job loss, privacy, and AI's potential to “surpass human skills.” (builtin.com)
- According to the company's website, more than 800 financial firms use AlphaSense, including some Fortune 500 corporations. (builtin.com)
External Links
How To
How do I start using AI?
You can use artificial intelligence by creating algorithms that learn from past mistakes. This can be used to improve your future decisions.
You could, for example, add a feature that suggests words to complete your sentence if you are writing a text message. It would take information from your previous messages and suggest similar phrases to you.
It would be necessary to train the system before it can write anything.
You can even create a chatbot to respond to your questions. You might ask "What time does my flight depart?" The bot will answer, "The next one leaves at 8:30 am."
Take a look at this guide to learn how to start machine learning.